Concurrency is working on multiple things at the same time. In Python, concurrency can be reached in several ways:
- With threading, by letting multiple threads take turns.
- With multiprocessing, we’re using multiple processes. This way we can truly do more than one thing at a time using multiple processor cores. This is called parallelism.
- Using asynchronous IO, firing off a task and continuing to do other stuff, instead of waiting for an answer from the network or disk.
- By using distributed computing, which basically means using multiple computers at the same time
Table of Contents
Python concurrency basics
Although Python concurrency can be difficult at times, it’s also rewarding and a great skill to have in your toolbelt. Let’s first start with some basic knowledge. Next, we’ll ask ourselves the question: do I really need (or want) concurrency, or are the alternatives a good enough solution for me?
The difference between threads and processes
So we already learned there are multiple ways to create concurrency in Python. The first and lightweight way is using threads.
A Python thread is an independent sequence of execution, but it shares memory with all the other threads belonging to your program. A Python program has, by default, one main thread. You can create more of them and let Python switch between them. This switching happens so fast that it appears like they are running side by side at the same time.
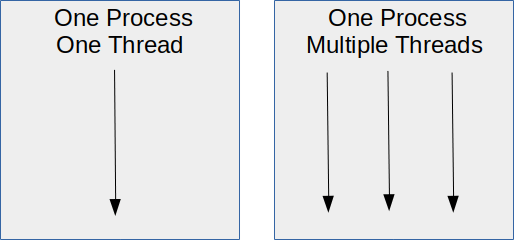
The second way of creating concurrency in Python is by using multiple processes. A process is also an independent sequence of execution. But, unlike threads, a process has its own memory space that is not shared with other processes. A process can clone itself, creating two or more instances. The following image illustrates this:
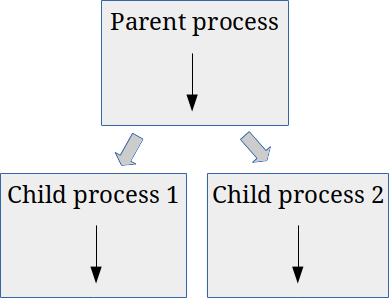
In this image, the parent process has created two clones of itself, resulting in the two child processes. So we now have three processes in total, working all at once.
The last method of creating concurrent programs, is using asynchronous IO, or asyncio for short. Asynchronous IO is not threading, nor is it multiprocessing. In fact, it is a single-threaded, single-process paradigm. I will not go into asynchronous IO in this chapter for now, since it’s somewhat new in Python, and I have the feeling that it’s not entirely there yet. I do intent to extend this chapter in the future to cover asyncio as well.
I/O bound vs CPU bound problems
Most software is I/O bound and not CPU bound. In case these terms are new to you:
- I/O bound software
- Software that is mostly waiting for input/output operations to finish, usually when fetching data from the network or from storage media
- CPU bound software
- Software that uses all CPU power to produce the needed results. It maxes out the CPU.
Let’s again look at the different ways of reaching concurrency in Python, but now from an I/O bound and CPU bound perspective.
Python concurrency with threads
While waiting for answers from the network or disk, you can keep other parts running using multiple threads. A thread is an independent sequence of execution. Your Python program has, by default, one main thread. But you can create more of them and let Python switch between them. This switching happens so fast that it appears like they are running side by side at the same time.
Unlike other languages, however, Python threads don’t run at the same time; they take turns instead. The reason for this is a mechanism in Python called the Global Interpreter Lock (GIL). In short, the GIL ensures there is always one thread running, so threads can’t interfere with each other. This, together with the threading library, is explained in detail on the next page.
The takeaway is that threads make a big difference for I/O bound software but are useless for CPU bound software. Why is that? It’s simple. While one thread is waiting for a reply from the network, other threads can continue running. If you make a lot of network requests, threads can make a tremendous difference. If your threads are doing heavy calculations instead, they are just waiting for their turn to continue. Threading would only introduce more overhead: there’s some administration involved in constantly switching between threads.
Python concurrency with asyncio
Asyncio is a relatively new core library in Python. It solves the same problem as threading: it speeds up I/O bound software, but it does so differently. I’m going to admit right away I was, until recently, not a fan of asyncio in Python. It seemed fairly complex, especially for beginners. Another problem I encountered is that the asyncio library has evolved a lot in the past years. Tutorials and example code on the web is often outdated.
That doesn’t mean it’s useless, though. It’s a powerful paradigm that people use in many high-performance applications, so I plan on adding an asyncio article to this tutorial in the near future.
Python concurrency with multiprocessing
If your software is CPU-bound, you can often rewrite your code in such a way that you can use more processors at the same time. This way, you can linearly scale the execution speed. This type of concurrency is what we call parallelism, and you can use it in Python too, despite the GIL.
Not all algorithms can be made to run in parallel. It is impossible to parallelize a recursive algorithm, for example. But there’s almost always an alternative algorithm that can work in parallel just fine.
There are two ways of using more processors:
- Using multiple processors and/or cores within the same machine. In Python, we can do so with the multiprocessing library.
- Using a network of computers to use many processors, spread over multiple machines. We call this distributed computing.
Python’s multiprocessing library, unlike the Python threading library, bypasses the Python Global Interpreter Lock. It does so by actually spawning multiple instances of Python. Instead of threads taking turns within a single Python process, you now have multiple Python processes running multiple copies of your code at once.
The multiprocessing library, in terms of usage, is very similar to the threading library. A question that might arise is: why should you even consider threading? You can guess the answer. Threading is ‘lighter’: it requires less memory since it only requires one running Python interpreter. Spawning new processes has its overhead as well. So if your code is I/O bound, threading is likely good enough and more efficient and lightweight.
Should I look into Python concurrency?
Before you consider concurrency in Python, which can be quite tricky, always take a good look at your code and algorithms first. Many speed and performance issues are resolved by implementing a better algorithm or adding caching. Entire books are written about this subject, but some general guidelines to follow are:
- Measure, don’t guess. Measure which parts of your code take the most time to run. Focus on those parts first.
- Implement caching. This can be a big optimization if you perform many repeated lookups from disk, the network, and databases.
- Reuse objects instead of creating a new one on each iteration. Python has to clean up every object you created to free memory. This is what we call garbage collection. The garbage collection of many unused objects can slow down your software considerably.
- Reduce the number of iterations in your code if possible, and reduce the number of operations inside iterations.
- Avoid (deep) recursion. It requires a lot of memory and housekeeping for the Python interpreter. Use things like generators and iteration instead.
- Reduce memory usage. In general, try to reduce the usage of memory. For example: parse a huge file line by line instead of loading it in memory first.
- Don’t do it. Do you really need to perform that operation? Can it be done later? Or can it be done once, and can the result of it be stored instead of calculated over and over again?
- Use PyPy or Cython. You can also consider an alternative Python implementation. There are speedy Python variants out there. See below for more info on this.
PyPy
You are probably using the reference implementation of Python, CPython. Most people do. It’s called CPython because it’s written in C. If you are sure your code is CPU bound, meaning it’s doing lots of calculations, you should look into PyPy, an alternative to CPython. It’s potentially a quick fix that doesn’t require you to change a single line of code.
PyPy claims that, on average, it is 4.4 times faster than CPython. It does so by using a technique called just-in-time compilation (JIT). Java and the .NET framework are other notable examples of JIT compilation. In contrast, CPython uses interpretation to execute your code. Although this offers a lot of flexibility, it’s also very slow.
With JIT, your code is compiled while running the program. It combines the speed advantage of ahead-of-time compilation (used by languages like C and C++) with the flexibility of interpretation. Another advantage is that the JIT compiler can keep optimizing your code while it is running. The longer your code runs, the more optimized it will become.
PyPy has come a long way over the last few years and can generally be used as a drop-in replacement for both Python 2 and 3. It works flawlessly with tools like Pipenv as well. Give it a try!
Cython
Cython offers C-like performance with code that is written mostly in Python but makes it possible to compile parts of your Python code to C code. This way, you can convert crucial parts of an algorithm to C, which will generally offer a tremendous performance boost.
It’s is a superset of the Python language, meaning it adds extras to the Python syntax. It’s not a drop-in replacement like PyPY. It requires adaptions to your code and knowledge of the extras Cython adds to the language.
With Cython, it is also possible to take advantage of the C++ language because part of the C++ standard library is directly importable from Cython code. Cython is particularly popular among scientific users of Python. A few notable examples:
- The SageMath computer algebra system depends on Cython, both for performance and to interface with other libraries
- Significant parts of the libraries SciPy, pandas, and scikit-learn are written in Cython
- The XML toolkit, lxml, is written mostly in Cython